I recently had the opportunity to lead a thought-provoking techUK event discussing the use of Artificial Intelligence (AI) in the justice and public safety sectors. It was inspiring to be in a room with key players from the Police Digital Service, Crown Prosecution Service (CPS), and Ministry of Justice (MoJ), alongside innovative SMEs and larger suppliers. It turned out to be a true ‘market-shaping’ event with all attendees openly sharing their thoughts as we explored the future of data and AI.
Here are some of my takeaways from the event.
Why is AI progress so slow?
A major point of frustration for many in the room was the slow pace of progress on AI projects within public safety. What should take 6 weeks for a discovery phase or proof-of-concept (PoC) often stretches to 6 or 7 months. This delay appeared to be mainly down to a couple of issues.
- Lack of open APIs: The absence of open APIs in policing prevents the seamless flow of data. When combined with vendor lock-in, this restriction hampers information sharing between agencies and stifles the scalability of AI applications.
- Fragmented IT systems: We know that many police forces operate with their own IT systems, leading to a lack of standardisation. This fragmentation makes it difficult to integrate AI technologies across different forces, as there is no unified approach to data management.
Building a fail-learn-fast culture
To drive progress, I believe we need a cultural shift that embraces the concept of ‘failing fast’ safely and securely. This mindset encourages organisations to experiment with new ideas and strategies without the fear of failure. By adopting this approach, public safety organisations can quickly test AI initiatives and evaluate their effectiveness.
A great example shared during the event was an AI assistant developed to analyse data and improve handling of domestic violence calls. This initiative achieved a remarkable nearly 30% reduction in call time, allowing officers to respond more effectively and better support survivors of domestic abuse. This successful PoC is now being rapidly scaled to help with other types of calls. The key learning themes from this relate to being clear on the business problem to be solved, effectively managing business change, gaining trust and buy-in with the operational users.
The need for a strong data governance model
A major point of discussion was the need for a robust data governance model. As it stands now, while police forces do share data, the interoperability across local, regional, and national levels is far from seamless. For example, data often moves from local police to the CPS and then to the MoJ, but issues with data quality and flow can limit the effectiveness of these processes. I feel strongly that removing the silos and improving insights is crucial for better case management and decision-making, especially regarding the victim and offender journey.
So there’s a clear need to improve data literacy and skills, define secure data handling in the AI world, and ensure data flows smoothly between agencies. To my mind, an inter-agency data and AI strategy is the answer. The emphasis should be on setting standards for new suppliers around open but secure APIs and data schemas that allow seamless integration and quicker time to value.
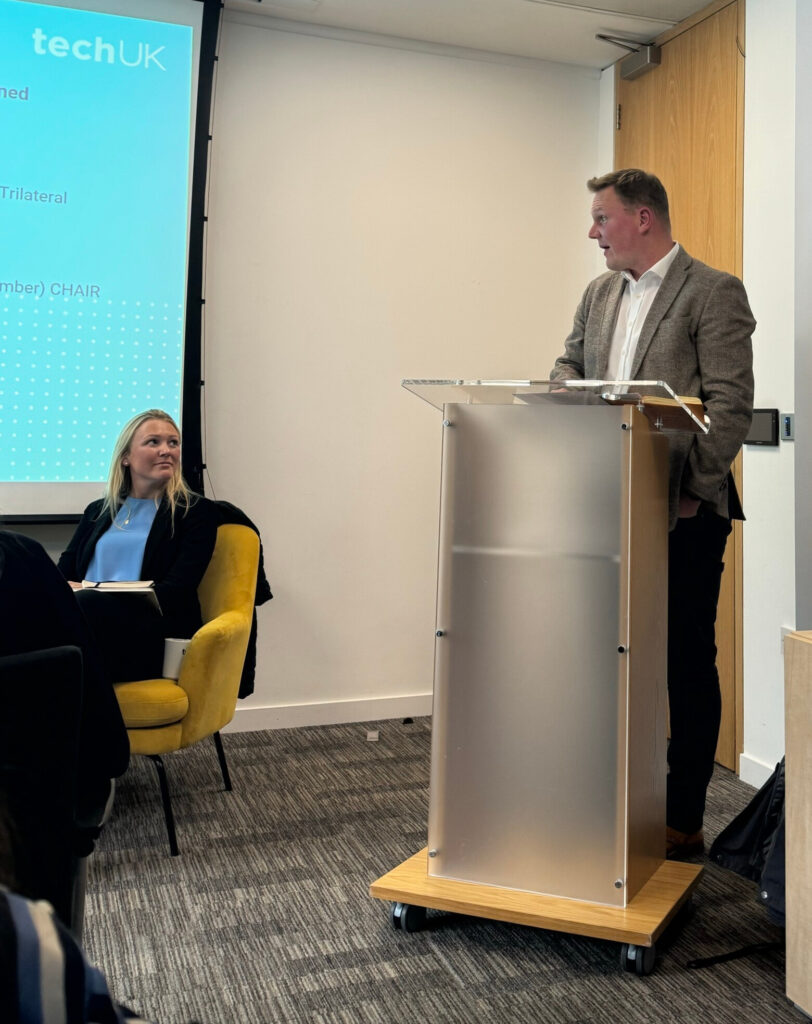
Rethinking traditional metrics and structures
I couldn’t help but reflect on the need to rethink how police forces are funded and evaluated. Traditional metrics often don’t incentivise innovation or support for digital projects. There was a strong consensus in the room that at a regional or national level we need to shift our focus to outcomes and innovation and how technology can support officer efficiency rather than solely focusing on a higher number of officers. To enable this, the current funding and governance model perhaps needs to change.
Enhancing transparency in AI decision-making
As AI continues to be integrated into policing and the justice system, we all discussed how important it is to understand the methodology behind AI-driven decisions. It’s not enough for AI to just provide answers. Decision-makers must know how it got to those answers. This transparency is a critical part of building trust with the public.
One of the exciting ideas discussed was fostering closer ties with academic institutions. I believe academic research plays a key role in exploring future use cases for AI and developing ethical frameworks. Collaborating with academia can help ensure that AI applications in policing and justice are both innovative, ethically sound and evidence based.
Everyone’s role in AI governance
Something else that struck me during the course of the day was the need for stronger leadership from senior executives in AI and data governance. Traditionally, technology decisions have been left to Chief Information Officers (CIOs) or Chief Data Officers (CDOs). However, given the ethical and societal implications of AI, it’s going to become essential for senior leaders across all departments to be involved in these decisions. AI is no longer just a technology issue; it’s a strategic concern that impacts the entire organisation.
Strong leadership is also vital for scaling AI projects. I noticed a significant gap between innovation and enterprise-level implementation, which indicates a lack of strategic enablement. Policymakers and senior leaders need to take a more active role in ensuring effective rollout and leverage national economies of scale.
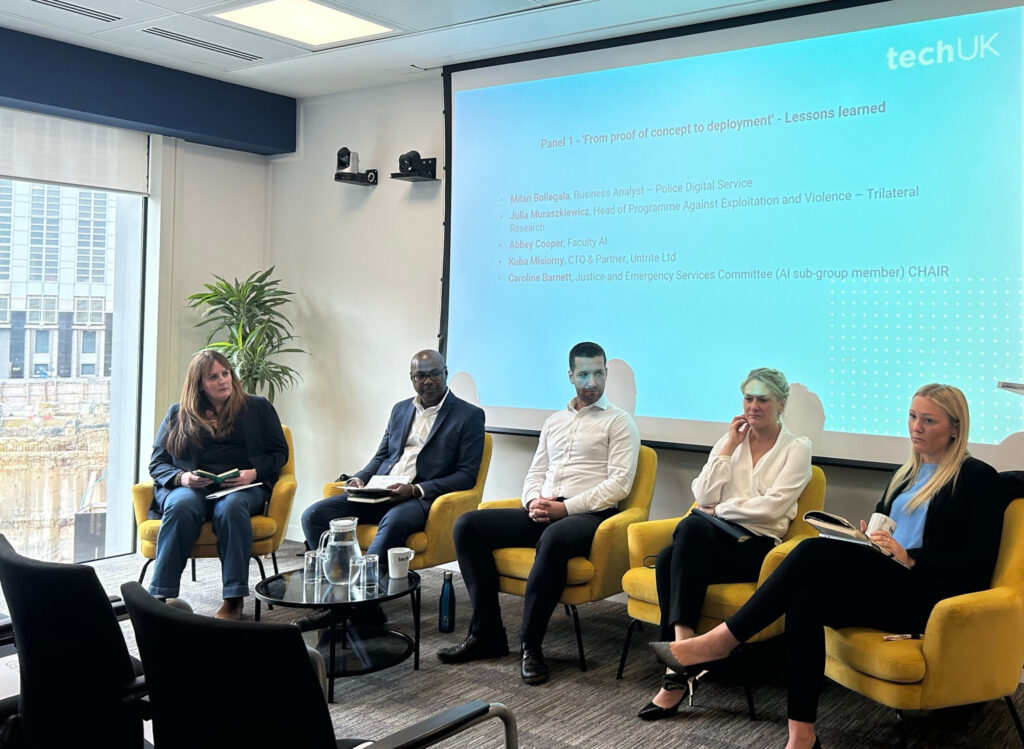
The path ahead
Attending this event emphasised the vast challenges ahead, but it also gave some clear direction on where we need to focus our attention. I left feeling that the future of policing will require bold decisions, innovative thinking, and a cultural shift towards sharing and transparency. I’m excited about the journey ahead and the possibilities that AI can bring to public safety and the justice system.
To find out more about my views on the challenges in this sector, take a look at my blog on ‘The top 3 ways to address challenges in the public safety sector’.
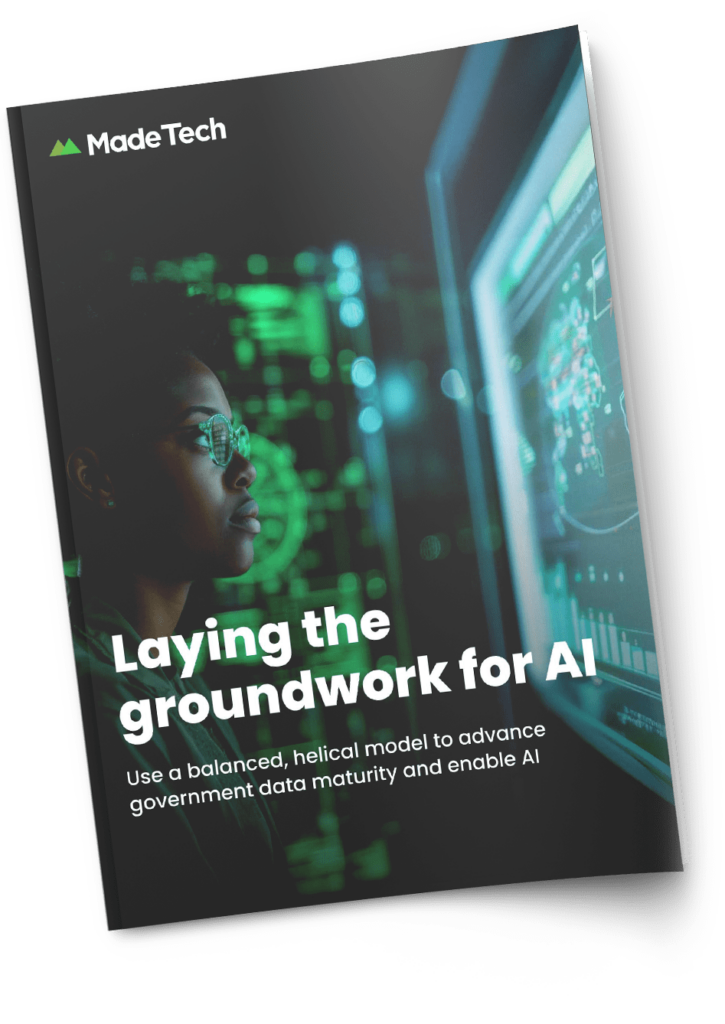
Laying the groundwork for AI
Unlock your AI potential: Discover your archetype, master the 3 pillars of data maturity, and learn from real-world transformations in our latest whitepaper, Laying the Groundwork for AI.